AI models begin to capture the whole central dogma of molecular biology
Read on to explore what this technology entails for future bioinformatics and how you can benefit from it already today
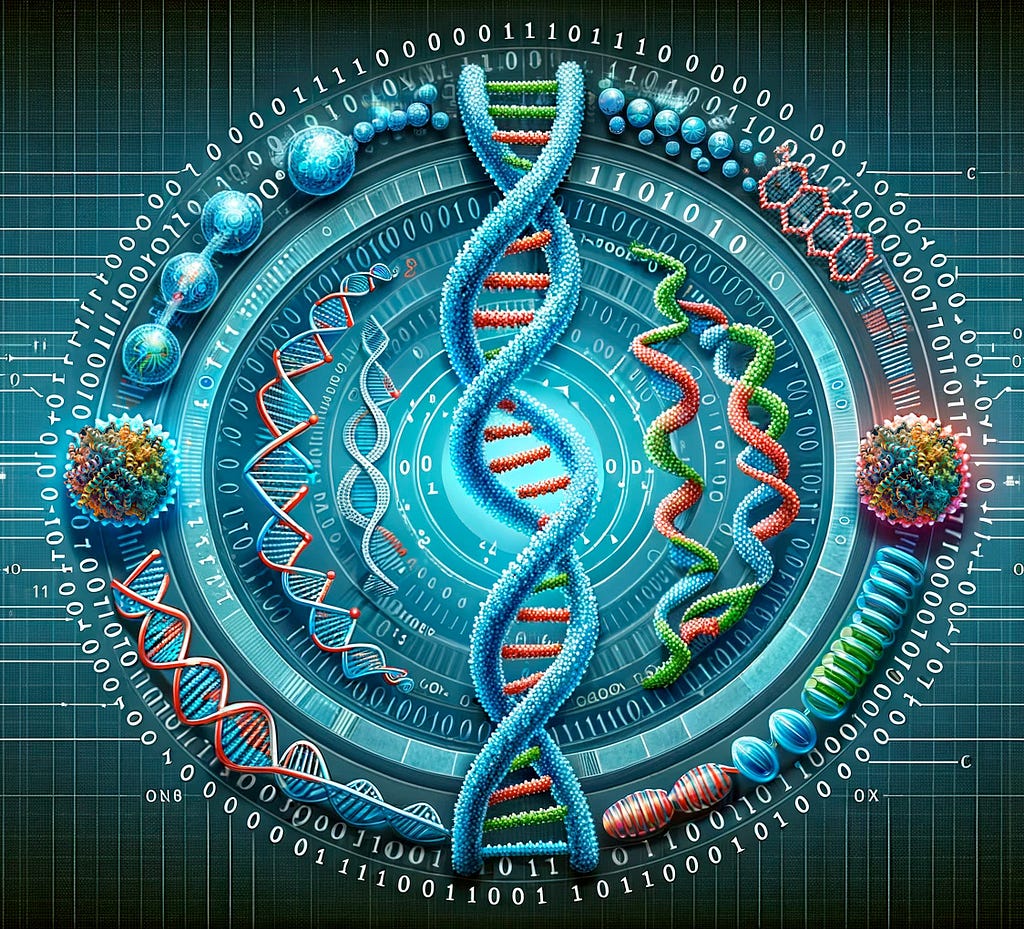
In a groundbreaking effort to what we can summarize as “have a computer understand the entirety of the central dogma of molecular biology and everything that arises from it”, a complex group of researchers have developed a new foundational multimodal model, Evo, that can parse and processes protein, DNA, and RNA sequences all at once, akin to how a multimodal AI model or an artificial general intelligence (AGI) can handle say text, video, and audio.
At least at its scale, Evo is first of its kind, and although just a newborn, it promises to soon open the door to exciting discoveries and new applications of AI to biology, much like AlphaFold 2, initially meant to predict protein structures, enabled tens of other applications and paved the way for whole new branches of AI applied to structural biology.
What Evo can do
Evo works as a foundational model designed to integrate information over long genomic sequences with single-nucleotide sensitivity, aiming to comprehend not just individual biomolecules and effects of mutations on them but also their complex interactions. This covers from literal physical interactions in multimolecular complexes to non-physical interactions between genes, regulatory elements, etc.
While Evo doesn’t aim to replace specialized models like say AlphaFold for structure prediction, its potential is noteworthy especially given that language-only models have proven capable of highly complex predictions. For example, Meta’s ESMfold has shown large capabilities in structure prediction and design, despite being essentially only trained on protein sequences-more precisely to predict masked amino acids, just like the large language models that power chatbots were trained to predict masked tokens that resemble word syllables.
Contrary to specialized models like AlphaFold, Evo’s potential lies in tasks that involve the interplay between different types of the core three biomolecules of biology’s central dogma: DNA, RNA and proteins. The central dogma states that DNA codes for RNA which codes for proteins, all interacting in complex forms from which all modern molecular biology emerges. This means processes, concepts and whole areas of research related to transcription, translation, gene regulation, epigenetics, genome evolution, natural and artificial gene editing, transposon biology, microRNA biology, and a long etcetera.
The preprint introducing Evo and its capabilities for multimodal integration includes examples showing how the model can, without fine tuning and without passing examples (i.e. zero-shot), assess gene essentiality, predict the effects of DNA mutations, generate plausible CRISPR-Cas systems by designing proteins and RNA molecules, generate DNA sequences at the genome scale i.e. with realistic signatures expected for real genes, and other tasks beyond the reach of current generative models focused on single molecular types.
While it is worth noting that none of the results was tested experimentally at detail (for example, no CRISPR-Cas system designed by Evo was actually produced and evaluated), this is essentially the same track that foundational work has taken in the years preceding models of actual practical use. This is a very first model of its type, and already shows large potential. We wouldn’t be too surprised if future versions of Evo, or perhaps some all-new models, can eventually achieve working predictions and generate functional designs, perhaps even get to model protein and nucleic acid structures and their complexes matching the accuracy of today’s specialized AI tools.
Developing Evo
Evo is a powerhouse with 7 billion parameters, capable of handling sequences up to 131 kilobases. Such large context window dwarfs the capabilities of any prior similar attempts, and is essential for Evo’s multimodal capabilities because it allows it to process long chunks of genomic sequences that include protein-coding regions, RNA molecules of different kinds and accessory DNA sequences all together. Only in this way can the relevant structural and genomic information arise in the internal mathematics of the model.
To make such large context window possible, Evo uses a hybrid architecture that combines attention and convolutional operators building on previous developments from part of the consortium. Importantly, this architecture allows integration of different convolutional layers and embeddings of varied size, which the team used to first pretrain Evo with a context length of 8,000 tokens that was then extended to 131,000 tokens with a gradual scaling that helped the model to learn and recognize sequence motifs effectively. All this, always keeping the single-nucleotide tokens as required for maximal resolution on training and inference.
An open dataset of genomic data
Another interesting point about how Evo was trained involves the compilation of a huge training dataset as required to train such large model and to render it truly foundational.
This dataset includes over 80,000 bacterial and archaeal genomes plus millions of predicted prokaryotic phage and plasmid sequences, totaling an outstanding 300 billion nucleotide tokens. All this data was assembled into a comprehensive dataset released together with Evo under the name of OpenGenome, that Evo’s developers promised to release soon for free use.
What Evo and similar technologies mean for us at Nexco
At Nexco we follow all new technologies very closely, discussing as a team their potential application in our pipelines and how to put them to your use. We bring our discussions to you through this blog, brainstorming ideas for the future -and in this case eager to see what Evo can do for us and for you.
Evo was developed by a consortium of public- and private-funded groups, which highlights the interest of the technology as a potential disruptor of fundamental and applied research. Foundational models, characterized by broad training on extensive datasets and adaptability for specific tasks of quite varied nature, signify a move towards more generalized intelligence. In this case, this is generalized intelligence about biology.
Just like we have witnessed in recent history with other AI models applied to science, it is clear that Evo’s potential for applications in biology has only just begun to peek through. Looking ahead, obvious next steps involve testing Evo’s knowledge and especially its predictions, to better define its practical capabilities. We can advance that once the model’s capabilities and limitations are well established, the next stage would be training larger models and including more genomes, in particularly to cover eukaryotic ones.
But like in the development of large language models for human-readable texts, and as Evo’s developers emphasize themselves, ethical issues arise that evoke a need for safety and responsible deployment protocols. For example, when the genomes were sourced to create the training dataset for Evo, pieces coding for obviously unsafe material such as viruses that infect eukaryotic hosts were excluded. But there are many sources of potentially harmful content in genomes, let alone the ethical issues of feeding AI models with genomic information from humans.
With its power and also limitations to be further unveiled, Evo could represent a stride towards integrating artificial intelligence with the intricate fabric of molecular biology, like scientists have never done before. As surely Evo will from now on be explored and scrutinized by the scientific community, we will follow this closely to see what transformative advancements it can definitely bring to bioinformatics and to deciphering and manipulating biology. We eagerly anticipate harnessing Evo’s potential to catalyze innovation in our pipelines and empower our community with new solutions.
Key references and links
Preprint presenting Evo:
Sequence modeling and design from molecular to genome scale with Evo
Blog post from one of Evo’s developers
Evo: Long-context modeling from molecular to genome scale
GitHub page for Evo
GitHub - evo-design/evo: DNA foundation modeling from molecular to genome scale
Related Posts
Nos locaux:
Nexco Analytics Bâtiment Alanine, Startlab Route de la Corniche 5A 1066 Epalinges, SwitzerlandAppelez-nous
+41 76 509 73 73Laissez-nous un message
contact@nexco.chN'hésitez pas à nous laisser un message
Nous nous ferons un plaisir de vous répondre et de trouver des solutions optimales à vos besoins.