Foundation Models for Biology to Support Bioinformatics
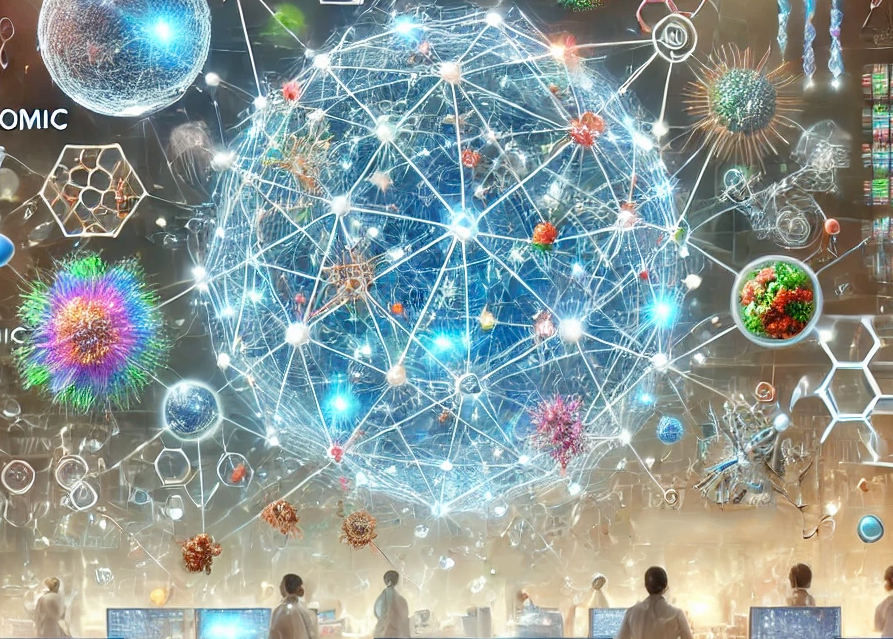
Imagine an AI system with so much “knowledge” about biology, that it can seamlessly integrate and analyze vast amounts of genomic, transcriptomic, proteomic, and other -omics data, even mixed together by being “aware” of the relationships between them. Such a system could be used for robust, cross-domain applications in bioinformatics: predicting the effects of genetic mutations, designing novel therapeutic interventions, and uncovering hidden biology.
This vision is slowly becoming a reality with the advent of larger and more complex foundational models trained on data that starts to escape specific domains, encompassing more biology and more (bio)chemistry. We recently covered an AI model called Evo that can process information at the core of biology’s central dogma. Late in 2023 we covered DeepMind’s AlphaMisssense model which harnesses the power of AlphaFold to predict the pathogenicity of missense mutations in proteins, thus connecting the worlds of genomics and protein structures. And just a month ago, the new AlphaFold 3 model was released which can connect protein structures to the structures of small molecules, thus completing the coverage from genomics on one end to the basics for drug discovery on the other.
Now, we present here three new foundational models called scFoundation, scGPT and Geneformer, reported throughout the 12 months in the journals Nature and Nature Methods, that are poised to push the boundaries of practical bioinformatics by harnessing the power of deep learning to process and interpret biological data of quite complex and cross-domain nature. Building on the success of pioneering models like scBERT, the new tools we present and discuss here offer higher accuracy and robustness in tasks ranging from cell type annotation to multi-omics data integration. In the rest of this blog post we will explore how these models could revolutionize bioinformatics and enable new discoveries and applications that were previously just unimaginable.
Three new Foundational Models to Assist Bioinformatics
Building on the success of previous models like scBERT, which pioneered the use of Transformer architectures in single-cell RNA sequencing (scRNA-seq) data analysis, new models are continuously pushing the boundaries of how AI can help bioinformatics. These models are designed to overcome the limitations of earlier methods, such as the reliance on curated marker gene lists, difficulty in leveraging latent gene-gene interactions, and improper handling of batch effects (although for this point in particular, we showed recently how sometimes simpler methods outperform complex AI models). With these and other advantages, the three new models presented here are in principle capable of providing more robust analyses and a more generalizable understanding of cellular biology.
Smart Transcriptomics With scFoundation
Two years ago, scBERT was introduced as a large-scale pretrained deep language model for cell type annotation of scRNA-seq data. By pretraining on massive unlabelled scRNA-seq data, scBERT attained superior performance in cell type annotation, novel cell type discovery, and robustness to batch effects. This model laid the groundwork for applying Transformer architectures to single-cell RNA-seq data analysis, providing robust and accurate annotations with gene-level interpretability.
Building on the foundation laid by scBERT, the scFoundation model represents a further leap in the application of AI to single-cell transcriptomics. With 100 million parameters covering about 20,000 genes and pretrained on over 50 million single-cell transcriptomic profiles, scFoundation excels in gene expression enhancement, tissue drug response prediction, and cell type annotation. Its architecture and its pretraining aware of read depths allow it to capture complex gene context relations effectively. This scalability and adaptability make scFoundation powerful for exploring interactions between genes, cellular states, and other features features hidden in scRNA-seq data.
Integrating Multi-Omics Data With scGPT
At its core, scGPT consists in a generative transformer architecture pretrained on scRNA sequences from over 33 million cells that cover a large fraction of the cellular heterogeneity across the human body. scGPT was then fine-tuned to perform downstream tasks from its embeddings. The authors found that specific fine-tuning procedures lead to models that excel at performing tasks such as annotating cell types, predicting responses to genetic perturbations, and integrating sc-RNA seq data from different batches or even containing various different kinds of -omics data, such as in the Multiome PBMC dataset of joint gene expression and chromatin accessibility measurements, or in the paired gene expression and protein abundance dataset from bone marrow mononuclear cells (BMMCs). Moreover, attention maps drawn from the architecture of the core and fine-tuned models appear to capture gene network patterns, further allowing the discovery of new biology. These last three example applications attest to the current and future potential of foundational models in allowing a more comprehensive and integrated understanding of genetics and biochemistry.
Exploring Network Biology Thanks to Transfer Learning
Geneformer is a context-aware, attention-based deep learning model designed to enable predictions in settings with limited data in network biology. It is pretrained on a vast corpus of around 30 million single-cell transcriptomes, which allowed it to gain a quite fundamental understanding of network dynamics. This pretrained model is then fine-tuned on a variety of downstream tasks on which it can perform well even with limited task-specific data.
In particular, the paper presenting Geneformer shows its remarkable capability in predicting dosage-sensitive disease genes, identifying candidate therapeutic targets, and enhancing predictive accuracy in chromatin and network dynamics. And in a stunning application to modeling a cardiomyopathy, Geneformer identified candidate therapeutic targets that, when experimentally inhibited, significantly improved cardiomyocyte contraction in a model of the disease. This represents a concrete example of how such these AI models can revolutionize biology and medicine, even in settings with limited data.
Why Intelligent AI Systems Matter
The development of intelligent AI systems that “understand” biology is not just a technological feat, but it also holds immense potential for advancing medicine, pharma, and our understanding of biology itself. More so, we pose, in the case of models that can integrate disparate pieces of biological information thus potentially capable of providing holistic views of cellular processes that might escape regular analyses.
This gains even more relevance as new techniques allow cheaper and wider capabilities to acquire vast amounts of data of different types, a hallmark of future medicine and biology as we discussed here.
Moreover, we can even imagine that a future system capable of understanding chemistry and protein biophysics on top of -omics data could be used to better predict the effects of genetic mutations, better identify potential drug targets, and even suggest new therapeutic interventions. Such predictive power would certainly accelerate the development of personalized medicine, where treatments are tailored to the genetic makeup of individual patients and their specific conditions.
Nexco’s View on the Future of Foundational Models for Biology
As scientists continue to develop and refine intelligent AI systems trained with larger and more varied datasets of biological data, we move closer to a future where computers can truly understand and thus help us to manipulate the complexities of biology. We are positive that eventually, models will become deep and versatile enough in the kinds of data they can handle, that they will connect different domains of expertise in novel ways; for example understanding genes not as not just mere letters in nucleic acid or protein sequence space that flag cellular states but actually as molecular entities that can then be actionable in terms of, for example, drug discovery. The implications of such kinds of technologies will be vast, impacting from disease diagnosis and disease treatment including computer-based personalization of therapies, to applications in biotechnology and to driving new discoveries in fundamental biology.
That’s why we at Nexco follow technological advancements closely, eager to see how the most modern AI models like scFoundation, scGPT, Geneformer, and others we have discussed in the past such as Evo, AlphaMissense, or the AlphaFold models, can be applied to our pipelines for your benefit.
Links
scFoundation:
Large-scale foundation model on single-cell transcriptomics - Nature Methods
Geneformer:
Transfer learning enables predictions in network biology - Nature
scGPT:
Related Posts
Nos locaux:
Nexco Analytics Bâtiment Alanine, Startlab Route de la Corniche 5A 1066 Epalinges, SwitzerlandAppelez-nous
+41 76 509 73 73Laissez-nous un message
contact@nexco.chN'hésitez pas à nous laisser un message
Nous nous ferons un plaisir de vous répondre et de trouver des solutions optimales à vos besoins.