Spatial Transcriptomic Data Analysis: A Beginner’s Guide
Essential Strategies for Effective Spatial Transcriptomic Data Analysis
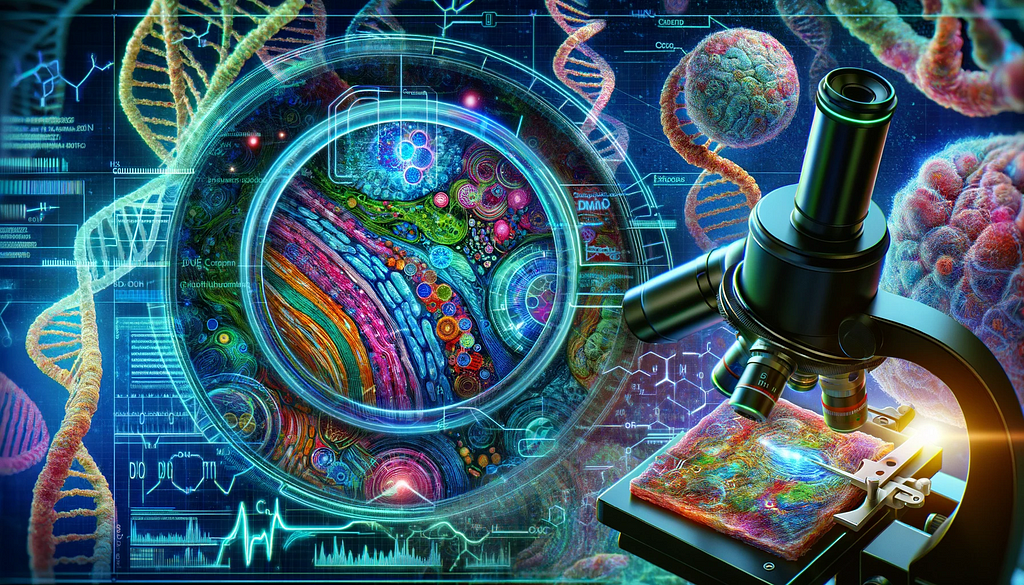
Analyzing high-dimensional spatial transcriptomic data appropriately and efficiently is an absolute must for any spatial transcriptomic experiment to produce accurate, robust, and biologically meaningful results. But, for many researchers, spatial transcriptomic data analysis remains challenging due to the sheer scale and complexity of data generated, not to mention the vast number of analysis options available. So, in the second installment of our spatial transcriptomic series, we provide some key considerations to help you get to grips with that all-important data analysis.
Spatial transcriptomic data analysis for imaging- or next-generation sequencing-based technologies
The first thing to consider is which spatial transcriptomic technique you used.
Some analysis pipelines are more appropriate for specific techniques than others, so selecting the methods that get the maximum information from your data while addressing your research question is vital (1).
There are two main spatial transcriptomic approaches — imaging-based and next-generation sequencing (NGS)-based — each requiring different initial pre-processing steps (2). You can learn more about these different technologies in the first article of our series.
Pre-processing image-based data
Imaging-based spatial transcriptomic techniques using single-molecule fluorescent in-situ hybridization (smFISH) or in situ sequencing (ISS) rely on multiple rounds of hybridization of base-detecting fluorophores to mRNA molecules, followed by successive imaging on a microscope to detect optical signatures of gene expression (2).
This process generates terabytes of raw data consisting of thousands of images of fluorescent spots that aren’t much use individually. To make sense of these spots, researchers must pre-process these raw images to convert them into easy-to-interpret gene-spot matrices containing transcript counts per gene by spatial area (2).
Removal of background noise and alignment of successive hybridization images so that one spot in each represents the same transcript are both core pre-processing steps, often performed with classical tools such as top-hat filtering (2) or more advanced deep learning packages such as DeepCell (3). Where spatial single-cell read-outs are required, the detected RNA must be grouped into individual cells by cell segmentation methods such as Baysor (4) to produce gene-cell matrices.
Pre-processing NGS with spatial barcoding data
NGS with spatial barcoding methods, such as Visium from 10X Genomics , use slides arrayed with positionally barcoded oligonucleotides to capture poly-A mRNA molecules from overlayed and imaged tissue sections (2).
The mRNA barcoding strategy is similar to that used in single-cell RNA-seq, meaning distinct overlaps in pre-processing and downstream data analysis techniques. However, for Visium from 10X Genomics, pre-processing with out-of-the-box solutions like Space Ranger are good starting points for standard analyses to decipher positional barcodes and mRNA sequences with minimal user input.
Space Ranger aligns reads to the genome of interest, matches read barcodes to spatial locations in the tissue, and counts the number of transcripts at each location to generate gene-spot matrices for downstream analysis, ultimately providing spatially resolved transcriptome-wide read-outs. Many other tools are also available for bespoke pre-processing or other spatial transcriptomic platforms like Stereo-seq (5).
Downstream analyses for spatial transcriptomics. What’s your question?
The type of research questions it is possible to answer with spatial transcriptomics is endless.
For instance, how do certain cells or genes interact with other cells or genes in specific places in tissue (6)? Is there a difference in gene expression between malignant cells in tumor cores and tumor edges (7)? Are there gene expression gradients in human brain development (8)?
Performing the correct downstream analysis for your specific hypothesis will ultimately streamline your quest for answers.
Most downstream analyses generally work with gene-spot matrices generated during pre-processing as input, regardless of the spatial transcriptomic technology used (2). Thanks to this standardized format, most analyses can be performed with tools initially developed for single-cell RNA-seq analysis, such as the popular Seurat in R (9) or Scanpy in Python (10), with some modifications. Specialist tools for spatial downstream analyses are also becoming increasingly popular, such as Giotto in R (11).
Normalization
A starting point in downstream analyses for NGS-based methods is the normalization of data to account for differences in the number of reads captured per spot, which can be substantial across tissue sections, especially where cells are more densely or loosely packed. If uncorrected, misleading results might creep in. Specialist normalization algorithms such as sctransform in Seurat (12) are often the most appropriate approaches for tissue with variable cell densities.
Cell deconvolution and inference
Unlike some imaging-based methods, NGS-based methods such as Visium do not have single-cell spatial resolution, as more than one cell can contribute to each spot (13). So, suppose your hypothesis requires spatial information about mRNA expression in single cells. In this case, Visium data must be deconvolved to identify and quantify the contribution of mRNA from each cell type in a capture spot (14).
Complex algorithms can infer this information. However, integration with a ‘ground truth’ scRNA-seq dataset is often the best course of action if available (14). Combining with scRNA-seq data can also be helpful if you think a gene wasn’t detected due to sensitivity issues and is used to impute missing data (14). Seurat (15) and Giotto (11) both provide robust deconvolution and inference methods.
Finding transcriptionally defined spatial regions and spatially variable genes
The goal of many spatial transcriptomic analyses is to identify transcriptomically similar areas of tissue and group these into features such as tissue domains (2). Some algorithms, such as XFuse (16) or stLearn (17), combine histology images with gene expression data via deep learning to infer gene expression between spots in an array or clusters. Seurat (15) and Giotto (11) also implement methods to identify regional clusters and spatially variable genes, with additional options in Giotto and stLearn to identify cell-type enrichment in Visium spots, gene coexpression, and cell-type colocalization, among many other capabilities.
Cell-cell interactions
Cell-cell interactions are fundamental to the correct development or functioning of tissues. Various methods can now interrogate ligand-receptor gene expression defined in gene relationship databases but in the spatial context (18).
Algorithms such as COMMOT (19), CellPhoneDB v3 (20), and Giotto (11), among others, all provide researchers with valuable cell-cell interaction data.
How Nexco Analytics can help with your spatial transcriptomic data analysis
Ultimately, a vast number of analyses can be performed with spatial transcriptomic data, and the most appropriate tool for the job largely depends on your research question, biological hypothesis, and the spatial transcriptomic technology used.
With our extensive spatial transcriptomic analysis expertise, at Nexco Analytics, we can help take the headache out of deciding which is the most appropriate analysis tool for the job while performing the analyses with streamlined, data-secure processes to ensure robust, reliable, and biologically meaningful results that drives your research forwards.
Please get in touch with us to see how we can help.
References
(1) Yue L, Liu F, Hu J, Yang P, Wang Y, Dong J, Shu W, Huang X, Wang S. A guidebook of spatial transcriptomic technologies, data resources and analysis approaches. Computational and Structural Biotechnology Journal. 2023 Jan 16.
(2) Moses L, Pachter L. Museum of spatial transcriptomics. Nature Methods. 2022 May;19(5):534–46.
(3) Bannon D, Moen E, Schwartz M, Borba E, Kudo T, Greenwald N, Vijayakumar V, Chang B, Pao E, Osterman E, Graf W. DeepCell Kiosk: scaling deep learning–enabled cellular image analysis with Kubernetes. Nature methods. 2021 Jan;18(1):43–5.
(4) Petukhov V, Xu RJ, Soldatov RA, Cadinu P, Khodosevich K, Moffitt JR, Kharchenko PV. Cell segmentation in imaging-based spatial transcriptomics. Nature biotechnology. 2022 Mar;40(3):345–54.
(5) Chen A, Liao S, Cheng M, Ma K, Wu L, Lai Y, Qiu X, Yang J, Xu J, Hao S, Wang X. Spatiotemporal transcriptomic atlas of mouse organogenesis using DNA nanoball-patterned arrays. Cell. 2022 May 12;185(10):1777–92.
(6) Cang Z, Zhao Y, Almet AA, Stabell A, Ramos R, Plikus MV, Atwood SX, Nie Q. Screening cell–cell communication in spatial transcriptomics via collective optimal transport. Nature Methods. 2023 Feb;20(2):218–28.
(7) Arora R, Cao C, Kumar M, Sinha S, Chanda A, McNeil R, Samuel D, Arora RK, Matthews TW, Chandarana S, Hart R. Spatial transcriptomics reveals distinct and conserved tumor core and edge architectures that predict survival and targeted therapy response. Nature Communications. 2023 Aug 18;14(1):5029.
(8) Zhong S, Wang M, Huang L, Chen Y, Ge Y, Zhang J, Shi Y, Dong H, Zhou X, Wang B, Lu T. Single-cell epigenomics and spatiotemporal transcriptomics reveal human cerebellar development. Nature Communications. 2023 Nov 22;14(1):7613.
(9) Satija R, Farrell JA, Gennert D, Schier AF, Regev A. Spatial reconstruction of single-cell gene expression data. Nature biotechnology. 2015 May;33(5):495–502.
(10) Wolf FA, Angerer P, Theis FJ. SCANPY: large-scale single-cell gene expression data analysis. Genome biology. 2018 Dec;19:1–5.
(11) Dries R, Zhu Q, Dong R, Eng CH, Li H, Liu K, Fu Y, Zhao T, Sarkar A, Bao F, George RE. Giotto: a toolbox for integrative analysis and visualization of spatial expression data. Genome biology. 2021 Dec;22:1–31.
(12) Hafemeister, C. and Satija, R., 2019. Normalization and variance stabilization of single-cell RNA-seq data using regularized negative binomial regression. Genome biology, 20(1), p.296.
(13) Ståhl PL, Salmén F, Vickovic S, Lundmark A, Navarro JF, Magnusson J, Giacomello S, Asp M, Westholm JO, Huss M, Mollbrink A. Visualization and analysis of gene expression in tissue sections by spatial transcriptomics. Science. 2016 Jul 1;353(6294):78–82.
(14) Li B, Zhang W, Guo C, Xu H, Li L, Fang M, Hu Y, Zhang X, Yao X, Tang M, Liu K. Benchmarking spatial and single-cell transcriptomics integration methods for transcript distribution prediction and cell type deconvolution. Nature methods. 2022 Jun;19(6):662–70.
(15) Hao Y, Stuart T, Kowalski MH, Choudhary S, Hoffman P, Hartman A, Srivastava A, Molla G, Madad S, Fernandez-Granda C, Satija R. Dictionary learning for integrative, multimodal and scalable single-cell analysis. Nature Biotechnology. 2023 May 25:1–2.
(16) Bergenstråhle L, He B, Bergenstråhle J, Abalo X, Mirzazadeh R, Thrane K, Ji AL, Andersson A, Larsson L, Stakenborg N, Boeckxstaens G. Super-resolved spatial transcriptomics by deep data fusion. Nature biotechnology. 2022 Apr;40(4):476–9.
(17) Pham D, Tan X, Xu J, Grice LF, Lam PY, Raghubar A, Vukovic J, Ruitenberg MJ, Nguyen Q. stLearn: integrating spatial location, tissue morphology and gene expression to find cell types, cell-cell interactions and spatial trajectories within undissociated tissues. BioRxiv. 2020 May 31:2020–05.
(18) Liu Z, Sun D, Wang C. Evaluation of cell-cell interaction methods by integrating single-cell RNA sequencing data with spatial information. Genome Biology. 2022 Dec;23(1):1–38.
(19) Cang Z, Zhao Y, Almet AA, Stabell A, Ramos R, Plikus MV, Atwood SX, Nie Q. Screening cell–cell communication in spatial transcriptomics via collective optimal transport. Nature Methods. 2023 Feb;20(2):218–28.
(20) Efremova M, Vento-Tormo M, Teichmann SA, Vento-Tormo R. CellPhoneDB: inferring cell–cell communication from combined expression of multi-subunit ligand–receptor complexes. Nature protocols. 2020 Apr;15(4):1484–506.
Related Posts
Our location
Nexco Analytics Bâtiment Alanine, Startlab Route de la Corniche 5A 1066 Epalinges, SwitzerlandGive us a call
+41 76 509 73 73Leave us a message
contact@nexco.chDo not hesitate to contact us
We will answer you shortly with the optimal solution to answer your needs