AI-Powered Protein Design Can Now Understand All Kinds of Biomolecules

Computational protein design is advancing at a fast pace after the cascade of developments in deep learning applied to molecular systems triggered by Deepmind’s release of its AlphaFold 2 model back in 2020. Yet, a significant challenge remains: the existing AI models are quite limited in their ability to incorporate non-protein elements into the design process, limiting their versatility. A recent study from our neighbors at EPFL introduces a method that addresses this limitation head-on: the Context-aware Amino acid Recovery from Backbone Atoms and heteroatoms, CARBonAra. Read on as we present this new tool in the context of existing alternatives and of related work, discussing along the way the field of computational protein design, its applications and challenges, and the still important role of human expert intervention, all of which we offer at Nexco.
A New Era in Protein Design
CARBonAra leverages the power of geometric deep learning through a transformer of atomic coordinates trained by directly relating protein sequences with nothing more than the atomic coordinates and element names of the atoms that make up the molecular systems. By not relying on any amino acid-specific parametrizations, surface calculations, or any other initial setups or precalculations, CARBonAra is fast and flexible, and it can consider the molecular environment surrounding a protein natively and for absolutely any kind of molecule type. Thus, it can run protein sequence design constrained by any kind of molecular context — of course as long as the molecules of the context are represented in experimental structures of the Protein Data Bank, from which it was trained.
The paper presenting CARBonAra here in Nature Communications proves how it can understand molecular contexts upon protein design and how this enhances the recovery of catalytic sites, metal binding sites, and other sequence features that go beyond the requirements for a protein structured with the expected fold. Besides, the paper shows hands-on in the wet lab how CARBonAra can design highly thermostable, catalytically active enzymes, demonstrating this with the generation of a whole subfamily of beta-lactamase enzymes. In nature, bacteria produce these enzymes to hydrolyze beta lactam antibiotics and thus render them inactive, effectively becoming resistant to them which is we could say a negative functionality; however, thermostabilized lactamases are promising for the inactivation of beta-lactam antibiotics from wastewaters, and could well find other applications. More broadly, thermostabilized proteins have huge market value in several manufacture and biotechnological industries, as we have explored previously in our success stories optimizing enzyme stability through expert analysis.
How CARBonAra works
At its core, CARBonAra is built on a geometric transformer that processes atomic coordinates and element names to predict the most likely amino acids at each position in a protein sequence. Given a target protein structure together with its interacting ligands, ions, nucleic acids and other proteins, the output from CARBonAra is essentially much the same as that from other methods like ProteinMPNN: a position-specific scoring matrix from which sequences are then sampled for design. However, alternative methods such as ProteinMPNN can only tract protein molecules, disregarding non-protein elements. In contrast, CARBonAra recognizes the other molecular entities and takes them into account upon calculation of the position-specific scoring matrix, thus reaching higher accuracy in the prediction of residues close to interfaces and being more adaptable to different use cases. Besides, CARBonAra runs faster than other methods given the rather simple nature of the computations involved in the geometric transformer.
The power of novel AI-based “all-atom” protein modeling and design tools
While CARBonAra isn’t the first model capable of understanding molecules other than proteins, it is the first peer-reviewed model that can apply this understanding to protein design. We covered earlier tools like RoseTTAFold-AllAtoms from the Baker lab and AlphaFold 3 from Deepmind here and here, which can handle all kinds of molecules when it comes to predicting structures, for example for proteins complexed to nucleic acids or bound to lipids, ions and small molecules such as drugs. From the Baker lab, LigandMPNN can account for non-protein contexts upon protein design pretty much like CARBonAra, but is for the moment in evaluation (preprint here).
The potential applications of protein design tools like CARBonAra and the upcoming LigandMPNN are truly vast. In enzyme engineering, for instance, these new tools can help in the design of new variants of enzymes that are stable and functional at high temperatures — an essential feature for many industrial processes as we covered here. In biotechnology, the new tools would allow crafting of better, more proficient and selective enzymes and protein reagents. They could also help to craft protein therapeutics that are more effective and personalized, offering a promising alternative to traditional small molecule drugs and reshaping the future of medical treatments.
As CARBonAra’s developers discuss in their paper and advance with their mixed computational and experimental studies on lactamases, these tools could also have an impact on studies of molecular evolution. Namely, they could provide structural insights into how proteins evolve and adapt to different molecular environments in evolutionary trajectories that lead to bacterial resistance to antibiotics, cancer relapse, etc.
Harness the power of modern AI-based protein design with Nexco’s expert advice
While we at Nexco are excited about the capabilities of AI tools for protein design, we are well aware that human intervention and expertise are still critical to increase the chances of successful sequence designs. The first challenge shows up already before running your AI model of choice, as you have to carefully select and prepare the input protein structure — a procedure that requires deep knowledge of protein structure and biophysics. Then, once the program has generated the position-specific scoring matrix that measures the probability of finding each amino acid at each site, you need to sample actual sequences from it, and this is in itself still an open problem in the field of protein design. Finally, one will typically generate large numbers of sequences that need to be scored with computational methods to rank different variants and submit to experimental validation a small number of them — again a step that requires practical knowledge about protein structure and biophysics.
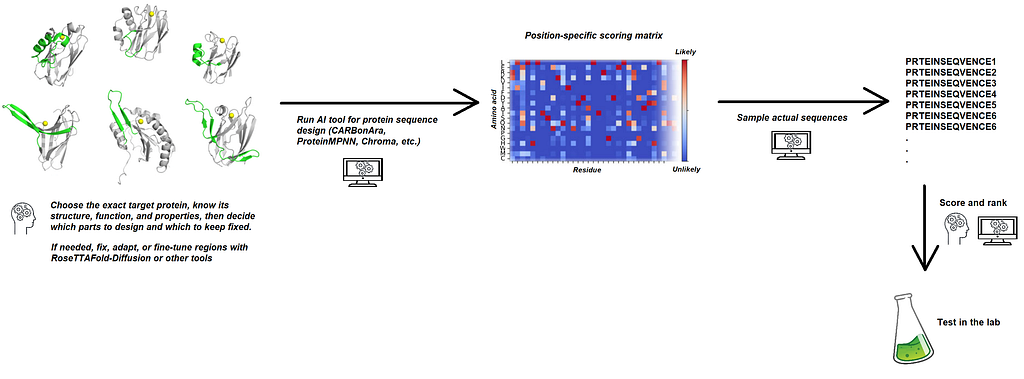
At Nexco we count both with computer experts who can setup and run the different pipelines required at each stage of the sequence generation, sampling and scoring procedures, and with experts in structural biology who can overview the process and act at the critical points where years of hands-on work on protein structures are critical.
With all this expertise combined and with our continuous update on the latest literature, we can help you leverage these powerful tools to design proteins that meet your specific needs.
References
CARBonAra paper at Nature Communications:
Context-aware geometric deep learning for protein sequence design - Nature Communications
Related Posts
Our location
Nexco Analytics Bâtiment Alanine, Startlab Route de la Corniche 5A 1066 Epalinges, SwitzerlandGive us a call
+41 76 509 73 73Leave us a message
contact@nexco.chDo not hesitate to contact us
We will answer you shortly with the optimal solution to answer your needs